Abstract
Paclitaxel is often used in combination with small molecule kinase inhibitors to enhance antitumor efficacy against various malignancies. Because paclitaxel is metabolized by CYP2C8 and CYP3A4, the possibility of drug–drug interactions mediated by enzyme inhibition may exist between the combining agents. In the present study, a total of 12 kinase inhibitors were evaluated for inhibitory potency in human liver microsomes by monitoring the formation of CYP2C8 and CYP3A4 metabolites simultaneously. For reversible inhibition, nilotinib was found to be the most potent inhibitor against both CYP2C8 and CYP3A4, and the inhibition potency could be explained by strong hydrogen binding based on molecular docking simulations and type II binding based on spectral analysis. Comparison of Ki values revealed that the CYP2C8 pathway was more sensitive toward some kinase inhibitors (such as axitinib), while the CYP3A4 pathway was preferentially inhibited by others (such as bosutinib). Pathway-dependent inactivation (time-dependent inhibition) was also observed for a number of kinase inhibitors against CYP3A4 but not CYP2C8. Further studies showed that axitinib had a KI of 0.93 μM and kinact of 0.0137 min−1, and the observed inactivation toward CYP3A4 was probably due to the formation of reactive intermediate(s). Using a static model, a reasonably accurate prediction of drug–drug interactions was achieved by incorporating parallel pathways and hepatic extraction ratio. The present results suggest that potent and pathway-dependent inhibition of CYP2C8 and/or CYP3A4 pathways by kinase inhibitors may alter the ratio of paclitaxel metabolites in vivo, and that such changes can be clinically relevant as differential metabolism has been linked to paclitaxel-induced neurotoxicity in cancer patients.
Introduction
Metabolism is an important mechanism that the body employs to eliminate xenobiotics, such as drugs. In the drug discovery and development arena, it is important to identify metabolic pathways and key enzymes involved in drug clearance, as metabolites produced by different enzymatic pathways may possess different activity or toxicity profiles. With the increasing use of combination therapies, particularly among anticancer agents, such as paclitaxel-based combinations, the potential for drug–drug interaction needs to be carefully examined to achieve optimal clinical results. For drugs with multiple metabolic pathways, it is beneficial to understand the sensitivity of each pathway to inhibition or induction because the selectivity toward a particular pathway may alter metabolite profiles, hence affecting efficacy and safety outcomes. Consequently, such information would help reduce the risk of drug–drug interactions and optimize the safety and effectiveness of combination therapies.
Paclitaxel (taxol) is a cytotoxic agent that is widely used for treating various tumor types, such as in breast, lung, and ovarian cancer. The drug is administered via constant-rate intravenous infusion in either a once every 3- or 1-week regimen. Paclitaxel treatment is often associated with toxicities such as myelosuppression and neurotoxicity that exhibit marked intersubject variability (Marupudi et al., 2007). Research has shown that the severity of paclitaxel-induced neurotoxicity is dose-dependent and may be related to its disposition mechanisms (Bergmann et al., 2011; Leskelä et al., 2011). Paclitaxel is metabolized by CYP2C8 to form 6α-hydroxypaclitaxel at the C6 position of the taxane ring and by CYP3A4 to form 3′-hydroxypaclitaxel at the phenyl C3′-position on the C13 side chain (Monsarrat et al., 1990; Walle et al., 1993). In human bile samples, 6α-hydroxypaclitaxel (∼13% of the dose) is about 4-fold higher than p-3′-hydroxypaclitaxel (∼3% of the dose), suggesting that CYP2C8 plays a major role in the metabolic clearance of paclitaxel in vivo (Monsarrat et al., 1993, 1998). In human liver microsomes (HLMs), it has been reported that levels of 6α-hydroxypaclitaxel are consistently higher (∼2.3-fold) than those of p-3′-hydroxypaclitaxel (Taniguchi et al., 2005), while a more extensive study using 47 individual HLMs showed that CYP3A4-mediated paclitaxel p-3′-hydroxylation could be more predominant in subjects receiving phenobarbital treatment (Sonnichsen et al., 1995). In addition to cytochrome P450 (P450)–mediated metabolic clearance, efflux transport mediated by P-glycoprotein (P-gp) may play a role in paclitaxel disposition (Sparreboom et al., 1997).
Pharmacokinetic variability of paclitaxel in patients has been thought to be associated with different expressions and activities of CYP2C8, CYP3A4, and P-gp, and numerous studies have been conducted to examine the role of polymorphisms in CYP enzymes and their relationship with intersubject variability in efficacy and neurotoxicity. Recent reports suggest that while CYP2C8*3 is associated with a higher rate of clinical responses, it is also correlated with an increased risk for neurotoxicity (Leskelä et al., 2011; Hertz et al., 2012, 2013). In addition, the CYP3A4*22 genotype has been shown to affect paclitaxel-induced neurotoxicity (de Graan et al., 2013). Furthermore, changes in the clearance of the CYP2C8 metabolite, 6α-hydroxypaclitaxel, is correlated to ABCB1 polymorphism and P-gp activity (Fransson et al., 2011). These findings indicate that P450-mediated paclitaxel metabolism and possibly specific metabolites are important factors affecting its clinical efficacy and toxicity and that drug–drug interactions caused by P450 inhibition may have important clinical implications for combination therapies.
Paclitaxel-based combination therapies are often used to evaluate the newer generation of agents such as kinase inhibitors to maximize antitumor efficacy (see Table 1 for more information). Although numerous studies have been conducted for such combination therapies, drug–drug interactions between paclitaxel and kinase inhibitors have not been fully examined in detail. Therefore, it is of clinical relevance to investigate the inhibition of P450-mediated paclitaxel metabolism by kinase inhibitors and evaluate drug-interaction potentials between the combining agents. In the present report, a total of 12 kinase inhibitors representing different structures (Supplemental Table 1) were obtained commercially, and their inhibitory potency against CYP2C8- and CYP3A4-mediated paclitaxel hydroxylation was examined in HLM. Data indicate that the inhibition of paclitaxel metabolism is pathway dependent and that different metabolic pathways exhibit certain sensitivities toward those kinase inhibitors, suggesting that the formation and ratio of paclitaxel metabolites can be altered in the presence of kinase inhibitors. Such findings would provide valuable information for the understanding of metabolic interactions between the combining agents, thus leading to further optimization of paclitaxel-based combination therapies.
Summary of key information for selected kinase inhibitors
Materials and Methods
Paclitaxel and docetaxel were purchased from Sigma-Aldrich (St. Louis, MO). NADPH was purchased from Roche (Basel, Switzerland). HLM (cat. no. 452161, pooled from 20 different organ donors), 6α-hydroxypaclitaxel, and p-3′-hydroxypaclitaxel were purchased from BD Gentest (Woburn, MA). Bactosomes containing human CYP3A4 and CYP2C8 were purchased from Cypex (Dundee, UK). Small molecule kinase inhibitors (>99% purity by high-performance liquid chromatography) were obtained from ChemBest Bioscience, Inc. (Shanghai, People’s Republic of China) and StruChem Co. Ltd. (Suzhou, People’s Republic of China). Disodium hydrogen phosphate and sodium dihydrogen phosphate were obtained from SinoPharm Chemical Reagent Co. (Shanghai, People’s Republic of China). All other reagents and chemicals were of analytical grade and of the highest quality available commercially.
Incubations with HLMs.
CYP2C8- and CYP3A-mediated paclitaxel hydroxylation in HLM were evaluated based on the formation of 6α-hydroxypaclitaxel and p-3′-hydroxypaclitaxel simultaneously. The linearity of metabolite formation as a function of time and protein concentration was first examined. The kinetic parameters were also obtained for the two hydroxylation pathways. The final experimental procedures were set as follows: the incubation mixture (200 μl) contained phosphate buffer (100 mM at pH 7.4), 1 mM NADPH, 0.2 mg/ml HLM, and the substrate paclitaxel (5 μM, ∼Km), and the reaction was initiated with the addition of NADPH. After 10 minutes of incubation, a 200-μl aliquot of cold acetonitrile containing the internal standard (docetaxel) was added to the reaction mixtures to quench the reaction. The samples were then centrifuged, and aliquots of 10 μl of the supernatants were subjected to liquid chromatography–tandem mass spectrometry (LC-MS/MS) analysis. Levels of 6α-hydroxypaclitaxel and p-3′-hydroxypaclitaxel were quantified as described herein.
Inhibition of Paclitaxel Hydroxylation by Kinase Inhibitors in HLMs.
Evaluation of the sensitivity of CYP2C8- and CYP3A-mediated paclitaxel hydroxylation toward a given inhibitor was performed by monitoring the formation of 6α-hydroxypaclitaxel and p-3′-hydroxypaclitaxel simultaneously in the same incubation. For reversible inhibition, Ki values were determined using the substrate paclitaxel at concentrations of 2.5, 5, and 10 μM, with inhibitor concentrations ranging from 0 to 100 μM. At the end of incubations, samples were prepared as described previously, and the levels of 6α-hydroxypaclitaxel and p-3′-hydroxypaclitaxel were quantified.
For the time-dependent inhibition component of this assay, kinase inhibitors at a concentration of 10 μM were first incubated with HLMs in the presence of NADPH. After a 30-minute preincubation, enzyme activities were determined with the addition of paclitaxel for CYP2C8 and CYP3A4 simultaneously. For compounds that demonstrated a strong reversible inhibition, a 20-fold dilution was performed after the 30-minute preincubation. After another 10 minutes of the incubation, the reaction mixtures were processed, and the samples were subjected to LC-MS/MS analysis for metabolite formation. Separately, enzyme activities without preincubation were determined with coincubation of paclitaxel and a given kinase inhibitor for 10 minutes in the presence of NADPH. By comparing the percentage of enzyme activity remaining between the above incubation settings, the time-dependent inhibition could be identified. Because the time-dependent inhibition of CYP3A4 using midazolam as a probe substrate has been reported for several kinase inhibitors and kinetic parameters are readily available (Kenny et al., 2012), the inactivation parameters of CYP3A4 using paclitaxel p-3′-hydroxylation were determined for axitinib only.
In brief, axitinib at concentrations of 0.3125, 0.625, 1.25, 2.5 and 5 μM was incubated with HLMs (4 mg/ml). At specified time points, the aliquots of the reaction mixture were transferred into a different set of tubes containing paclitaxel (5 μM) and the rest of the components including NADPH as described previously. This step resulted in a 20-fold dilution for the initial reaction mixture. After a further 10 minutes of incubation, the reaction mixtures were processed, and the samples were analyzed for the levels of p-3′-hydroxypaclitaxel.
Identification of Axitinib-Glutathione Adducts in HLMs.
Axitinib (10 μM) was incubated with HLM (1 mg/ml), glutathione (5 mM), and phosphate buffer (100 mM, pH 7.4) in a final reaction volume of 200 μl. The mixture was warmed at 37°C for 5 minutes, and the reaction was initiated with the addition of NADPH (1 mM). Control samples without NADPH were also prepared. The reaction was terminated at specified time points by adding 200 μl of ice-cold acetonitrile. The resulting mixture was centrifuged at 13,000g (4°C) to precipitate the proteins, and a 300-μl aliquot of supernatant was collected and evaporated to dryness under a stream of nitrogen. The pellet was redissolved in 100 μl of the mobile phase, and a 10-μl aliquot of the reconstituted solution was subjected to mass spectrometry analysis.
LC-MS/MS Analysis.
Quantitation of CYP2C8 and CYP3A4 metabolites was achieved by a LC-MS/MS system consisting of an API4000 Qtrap mass spectrometer equipped with a turbo-V ionization source (Applied Biosystems, Concord, ON, Canada), two LC-20AD pumps with a CBM-20A controller, DGU-20A solvent degasser, and a SIL-20A autosampler (Shimadzu Scientific Instruments, Columbia, MD). An Agela Venusil XBP C18 column (50 × 2.1 mm; 5 μm particle size) was used to achieve high-performance liquid chromatography separation (Bonna-Agela Technologies, Tianjin, People's Republic of China). The column temperature was held at 40°C. Gradient elution at a flow rate of 0.3 ml/min was performed using the following mobile phase: A, acetonitrile:water (5:95, v:v) and B, acetonitrile:water (95:5, v:v).
For MS/MS quantitation, the API4000 Qtrap mass spectrometer was operated in the electrospray ionization positive mode with multiple reaction-monitoring to detect paclitaxel metabolites and the internal standard with a dwell time set to 100 milliseconds. The ion transitions monitored were 6α-hydroxypaclitaxel, 892.3 (M + Na) → 308.1; p-3′-hydroxypaclitaxel, 892.3 (M + Na) → 324.1; and docetaxel (IS), 830.2 (M + Na) → 549.4. After setting the mass transition and collision energy for each metabolite and the internal standard, all other parameters were optimized for the best sensitivity. Data were collected and processed using the Analyst 1.5.2 data collection and integration software (AB Sciex, Framingham, MA).
The identification of the axitinib-glutathione adduct was achieved using an ACQUITY ultra-performance liquid chromatography system coupled with a quadruple time-of-flight mass spectrometer (Waters Corp., Milford, MA) with an electrospray ionization source. The chromatographic separation was done on an ACQUITY BEH C18 column (2.1 mm × 100 mm ID., 1.8 μm). The mobile phase was a mixture of 5 mM ammonium acetate aqueous solution containing 0.05% formic acid (A) and acetonitrile (B). At a flow rate of 0.4 ml/min, the mobile phase was held at 5% B for 2 minutes, and then the gradient started from 5% to 35% B in 8 minutes and increased linearly to 99% B. After maintaining for 1 minute at 99% B, mobile phase B was returned back to 5% for column equilibration. MS detection was conducted in the negative ionization mode.
The major operating parameters for the quadruple time-of-flight mass spectrometer were set as follows: capillary voltage, 4 kV; source temperature, 100°C; desolvation temperature, 350°C; collision gas, argon; desolvation gas (nitrogen) flow rate, 800 l/h; data acquisition range, m/z 80–1000 Da; and data format, centroid. Data were acquired under the MSE mode, in which two separate scan functions were programmed independently with low and high collision energies (CEs). The mass spectrometer switched rapidly between these two functions during data acquisition. As a result, information on intact precursor ions as well as fragment ions was obtained from one liquid chromatography run. In this study, one scan function used a low CE setting (5 eV of trap CE and 3 eV of transfer CE), and the other scan function used a high CE setting (ramped trap CE from 10 to 20 eV and 18 eV of transfer CE).
Data analysis and instrument control were performed using the MassLynx 4.1 software (Waters Corp.). MetaboLynx, a subroutine of the MassLynx software, was used to identify metabolite ions.
Molecular Docking Simulations of Kinase Inhibitors with CYP3A4 and CYP2C8.
To examine structural features for inhibitor-enzyme binding, molecular docking simulations were performed. The crystal structures of CYP3A4 (PDB ID 3UA1) (Sevrioukova and Poulos 2012) and CYP2C8 (PDB ID 2NNI) (Schoch et al., 2008) were obtained from the RCSB Brookhaven Protein Data Bank (Berman et al., 2000). The Protein Preparation module in Schrödinger 9.0 (Schrödinger, Portland, OR) was then used to remove the crystallographic water molecules, add hydrogen atoms, assign partial charges using the OPLS-2005 force field, assign protonation states, and minimize the structures (Kaminski et al., 2001). The minimization was terminated when the root-mean-square deviation reached a maximum value of 0.3 Å. The structures of the inhibitors were all sketched in Maestro and were minimized with Macromodel in Schrödinger. After that, the inhibitors were prepared with the Ligprep module, and the protonated states were generated at pH = 7.0 ± 2.0. Molecular docking simulations were performed with the Glide module in Schrödinger. Before docking, the binding grid box of size 10 Å × 10 Å × 10 Å was generated and centered on the ligand in the active pocket. In ligand docking, the extra-precision docking mode was adopted to generate the minimized pose with Glide program.
Spectral Analysis of the Binding of Kinase Inhibitors to CYP3A4 and CYP2C8.
To further investigate the binding type of kinase inhibitors with P450 enzymes, UV-visible absorbance of human CYP3A4 or CYP2C8 was recorded with a Thermo Scientific Multiskan Go spectrophotometer (Waltham, MA) in the split-beam mode. The experiments were performed by 1-nm step-scanning with a wavelength range encompassing 350 to 500 nm. Spectral analysis in the presence or absence of inhibitors was performed in matched microquartz cuvettes (2 mm × 10 mm, internal dimension) containing 0.2 μM CYP3A4 or CYP2C8 and 20% glycerol (v/v) in 50 mM tris-acetate buffer (pH 7.4) (Dahal et al., 2012). Stock solutions of kinase inhibitors were diluted to obtain a final concentration of 20 μM in the sample cuvettes, with the same amount of solvents added to the reference cuvette containing the same amount of enzymes (total volume of solvent added was <1%, v/v). Preliminary experiments using ketoconazole (a typical type II inhibitor) and nilotinib indicated that the mixture containing 0.2 μM P450 enzyme and 20 μM inhibitor yielded optimal spectral results. When applicable, values of spectral dissociation constant (Ks) were determined for kinase inhibitors with strong interactions (Isin and Guengerich, 2006).
Kinetic Data Analysis.
Inhibition potency (Ki) for each metabolic pathway by selected kinase inhibitors in the reversible inhibition setting was calculated using Enzyme Kinetics Modules of Sigma Plot 12.3 (Systat Software, San Jose, CA) based on the Dixon equations (Dixon, 1972). For the time-dependent inhibition of CYP3A4 by axitinib, kinetic parameters such as apparent KI and kinact were obtained using nonlinear regression (Prism 5.0; GraphPad Software, San Diego, CA). The average values of three separate incubations are presented.
Calculation of AUC Changes Based on In Vitro Inhibition Data.
Prediction of drug–drug interactions using in vitro inhibition data has been well described in the literature (Ito et al., 2005; Obach et al., 2006; Fahmi et al., 2008; Lu et al., 2008). The area under the curve (AUC) ratio (AUC′/AUC) of a victim drug is inversely related to the hepatic clearance ratio (CLH/CLH′) in the presence and absence of an inhibitor, assuming little or no renal clearance. Because parallel pathways (CYP2C8 and CYP3A4) are involved in paclitaxel metabolism, AUC changes can be simply described using equations as follows (Ito et al., 2005; Lu et al., 2008):
Equation 1 for reversible inhibition of both CYP2C8 and CYP3A4:
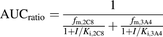
Equation 2 for reversible (CYP2C8) and time-dependent inhibition (CYP3A4):(2)where fm,2C8 or fm,3A4 is the fraction metabolized by CYP2C8 or CYP3A4, I is the inhibitor concentration (plasma Cmax is used in this case), Ki is the reversible inhibition constant, kinact is the inactivation rate constant, kdeg is the enzyme degradation rate constant, and KI is the inhibitor concentration that results in half-maximal enzyme inactivation.
Although considerable success has been achieved using the mathematical (static) models described above, these models assumed a very low hepatic extraction ratio (EH) and were best suited for orally administered victim drugs (Kirby and Unadkat, 2010). For comparison purposes and with the expectation to increase the accuracy for prediction, a recently described equation for intravenously administered drugs (Kirby and Unadkat, 2010) was adapted to calculate the AUC ratios in the presence or absence of a given kinase inhibitor. Equation 3 is for reversible inhibition (both CYP2C8 and CYP3A4):
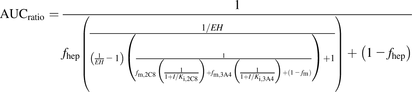
Equation 4 is for reversible (CYP2C8) and time-dependent inhibition (CYP3A4):(4)where EH is the hepatic extraction ratio of paclitaxel and fhep is the fraction of clearance subject to the hepatic blood flow limitation (Kirby and Unadkat, 2010).
Among variables that were used for the AUC ratio calculation, the Ki values were experimentally obtained for the 12 kinase inhibitors by monitoring CYP2C8- and CYP3A4-mediated paclitaxel hydroxylation. The values of kinact and KI for axitinib were also determined experimentally. The EH of paclitaxel after intravenous administration was calculated by using the equation:which is based on literature data (Smorenburg et al., 2003). For inhibitor concentration I, the plasma maximal concentrations at clinically relevant doses were used (Table 1). The literature value of kdeg (0.019 h−1) was assumed for CYP3A4 (Fahmi et al., 2008), and a value of 1 was assigned for fhep (Kirby and Unadkat, 2010). The fraction of the dose metabolized (fm) was estimated to be 0.963 based on paclitaxel human absorption, distribution, metabolism, and excretion (ADME) data (Monsarrat et al., 1993, 1998).
Results
Formation of 6α-Hydroxypaclitaxel and p-3′-Hydroxypaclitaxel in Pooled HLMs.
The activity of individual cytochrome P450 enzymes in HLM preparations varies markedly among different HLM sources. To maintain consistency and avoid complications due to source variability, the pooled HLM from the same batch were used throughout the present study. Under the current experimental conditions (0.2 mg/ml HLM and 5 μM paclitaxel), the formation of p-3′-hydroxypaclitaxel (CYP3A4) was linear over the 60-minute incubation period, and the formation of 6α-hydroxypaclitaxel (CYP2C8) was linear during the initial time points with the rate decreasing over time (data not shown). For subsequent experiments, the incubation time was set at 10 minutes to ensure the linear condition for the formation of both metabolites.
Kinetic studies revealed that Km and Vmax values for CYP3A4 and CYP2C8 pathways were 5.1 μM and 25.1 pmol/min/mg, and 5.2 μM and 222.1 pmol/min/mg, respectively. By comparing the ratio of Vmax/Km, the formation of 6α-hydroxypaclitaxel was almost 9 times faster than that of p-3′-hydroxypaclitaxel, suggesting that CYP2C8-mediated paclitaxel hydroxylation was more predominant in the current batch of pooled HLM. The Km and Vmax values observed in the present study were within 2-fold to 3-fold of the published results; this is not surprising given that laboratory-to-laboratory variability is often seen for in vitro metabolism studies (Polasek et al., 2004; Zhang et al., 2008). The differences in Km and Vmax values might be due to different HLM sources as well as the incubation conditions. It is also interesting to note that sigmoidal kinetics was observed for the p-3′-hydroxylpaclitaxel formation in HLM-H40 (Polasek et al., 2004).
Based on the above findings and the fm value from the literature (Monsarrat et al., 1993, 1998), the values of in vivo fm,2C8 and fm,3A4 were estimated to be 0.865 and 0.098, respectively, assuming that CYP2C8 and CYP3A4 were the only enzymes responsible for paclitaxel metabolism under current conditions. These fm values were used for the subsequent drug-drug interaction prediction.
Sensitivity of CYP2C8 and CYP3A4 Pathways to Reversible Inhibition.
As a well accepted probe substrate for CYP2C8, the formation of 6α-hydroxypaclitaxel is frequently used to evaluate the inhibitory potency of test compounds against CYP2C8 activity in HLM. Because paclitaxel is also metabolized by CYP3A4 and is often used in combination therapies, it was thus necessary to determine the inhibition of these two separate metabolic pathways by a given inhibitor simultaneously.
In the present study, the inhibitory potency of 12 kinase inhibitors was first determined in the reversible inhibition setting by monitoring the formation of 6α-hydroxypaclitaxel (CYP2C8) and p-3′-hydroxypaclitaxel (CYP3A4). Judging from the Ki values (Table 2), the studied kinase inhibitors displayed a wide range of inhibitory potency, among which nilotinib was the most potent inhibitor against both CYP2C8 (Ki = 0.10 μM) and CYP3A4 (Ki = 0.28 μM). This finding was consistent to a recent report (Kim et al., 2013). It was interesting to note that the selected kinase inhibitors displayed pathway-dependent inhibition toward CYP2C8- and CYP3A4-mediated paclitaxel hydroxylation. Based on the Ki ratios (CYP2C8 versus CYP3A4) and using 2-fold as an arbitrary cutoff, CYP2C8-mediated paclitaxel hydroxylation was more sensitive to inhibition by axitinib, lapatinib, nilotinib, and sorafenib, whereas CYP3A4-mediated paclitaxel hydroxylation was preferentially inhibited by bosutinib, dasatinib, erlotinib, pazopanib, and sunitinib. Afatinib, gefitinib, and imatinib displayed similar inhibitory potency against these two separate pathways (Table 2).
Inhibition potency of selected kinase inhibitors against the formation of 6α-hydroxypaclitaxel and p-3′-hydroxypaclitaxel
Sensitivity of CYP2C8 and CYP3A4 Pathways to Time-Dependent Inhibition.
The time-dependent inhibition of CYP2C8- and CYP3A4-mediated paclitaxel metabolism by kinase inhibitors was subsequently investigated. In this setting, a simpler approach was first adapted where the values of the percentage of enzyme activity remaining with or without a 30-minute preincubation of kinase inhibitors in the presence of NADPH were obtained at an inhibitor concentration of 10 μM. For inhibitors with a strong reversible inhibition at 10 μM, a 20-fold dilution assay was performed. Table 3 summarizes enzyme activity remaining with or without 30-minute preincubation in the presence of the studied kinase inhibitors.
Inhibition of the formation of 6α-hydroxypaclitaxel and p-3′-hydroxypaclitaxel by selected kinase inhibitors after a 30-minute preincubation
Based on the percentage of enzyme activity remaining, there was no obvious time-dependent inhibition of CYP2C8-mediated paclitaxel hydroxylation by those kinase inhibitors with the exception of afatinib, erlotinib, gefitinib, lapatinib, and sorafenib, which showed a modest time-dependent inhibition (10–30% activity changes).
In contrast, most of the selected kinase inhibitors (except sorafenib) showed strong time-dependent inhibition against CYP3A4-mediated paclitaxel metabolism, consistent with the literature reports (Li et al., 2009a; Teng et al., 2010; Filppula et al., 2012; Kenny et al., 2012). For the subsequent drug-drug interaction prediction, inactivation parameters (KI and kinact) of CYP3A4 by those kinase inhibitors were adapted from the literature reports (Li et al., 2009a; Teng et al., 2010; Filppula et al., 2012; Kenny et al., 2012). Because there were no data available for axitinib, we further investigated the kinetics and mechanisms of CYP3A4 inactivation by this drug.
As illustrated in Fig. 1, the inactivation of CYP3A4 by axitinib displayed characteristic time and concentration dependency. Kinetic analysis revealed that axitinib exhibited a KI of 0.93 μM and kinact of 0.0137 min−1 against CYP3A4-mediated paclitaxel hydroxylation. The kinact/KI ratio of axitinib against CYP3A4 was similar to that of dasatinib but smaller than that of nilotinib, suggesting an intermediate inhibition potency (Kenny et al., 2012).
Kinetic investigation on CYP3A4 inactivation by axitinib. (A) Time- and concentration-dependent inhibition of the formation of p-3′-hydroxypaclitaxel. (B) Nonlinear regression analysis of CYP3A4 inactivation by axitinib.
The CYP3A4 inactivation by axitinib might be caused by a reactive intermediate (epoxide) formed during the catalysis, as an axitinib-glutathione adduct was detected in the presence of NADPH. As shown in Fig. 2, two glutathione adducts were detected. Adduct-I had a retention time of 5.57 minutes with a molecular mass of 694.2, and adduct-II was eluted at 4.66 minutes with a molecular mass of 710.2, showing a difference of 16 Dalton. Additional experiments showed that adduct-I could be formed in the absence of NADPH, suggesting a direct conjugation of glutathione to axitinib. Based on the fragmentation pattern and accurate mass analysis, it was postulated that adduct-I (694.2188 → 565.171 → 387.127 → 356.087) was formed via a direct conjugation to the double bond, whereas adduct-II (710.2126 → 581.165 → 550.128 → 403.122 → 372.080) was formed at the same site, albeit after the formation of an epoxide intermediate in the presence of NADPH.
Identification of axitinib-glutathione adducts in human liver microsomes. (A) Extracted ion chromatograms of glutathione adducts in human liver microsomes. (B) Fragmentation patterns of axitinib-glutathione adducts.
Molecular Docking Simulations of Kinase Inhibitors with CYP3A4 and CYP2C8.
To examine the structural features that could explain the inhibitor-enzyme binding, we performed molecular docking simulations using the observed reversible Ki values. First, inhibitors bromergocryptine (08Y, a type I ligand) and montelukast (MTK) were redocked into CYP3A4 and CYP2C8, respectively, to evaluate the docking performance of Glide. According to the Glide docking results, the predicted structure of the 08Y-CYP3A4 complex agreed very well with 3UA1 crystal (Sevrioukova and Poulos 2012), but the predicted structure of MTK-CYP2C8 complex did not with 2NNI (Schoch et al., 2008). The root-mean-square deviation between the Glide-calculated pose and the experimentally-determined structure of 08Y in 3UA1 was 1.09 Å and that of MTK in 2NNI was 2.82 Å. It appeared that Glide could not accurately characterize some interactions between MTK and CYP2C8.
Based on the above observations, the studied kinase inhibitors were docked into the crystal structures of CYP3A4 and CYP2C8, respectively. The best conformation of each protein-ligand complex was determined by the lowest predicted binding free energies of the docked ligands. Figure 3 illustrates correlations between the best Glide extra-precision docking scores and the experimental Ki values of CYP3A4 and CYP2C8. It is of note that the docking scores for CYP3A4 exhibited a moderate correlation with experimental data (r2 = 0.64), but the docking scores of CYP2C8 showed a relatively lower linear correlation (r2 = 0.51). The results of the docking analysis suggest that different structural features of the studied kinase inhibitors might have different binding conformations, and thus further refinement is needed to characterize the interactions between the enzyme-inhibitor complexes.
Correlation between the Glide docking scores and the experimental logKi. (A) CYP3A4. (B) CYP2C8.
The binding mechanisms of two molecules (nilotinib and sunitinib) were further analyzed for their inhibitory potency. The predicted binding free energies of nilotinib and sunitinib were −9.81 and −7.11 kcal/mol for CYP3A4, and −9.94 and −6.10 kcal/mol for CYP2C8, respectively. Data suggest that nilotinib exhibited much higher binding affinities than sunitinib in both CYP3A4 and CYP2C8 systems, which is consistent with the inhibitory potency observed for nilotinib (Table 2). Figure 4A illustrates the binding mode of the CYP3A4-nilotinib complex. It can be noted that one of the three fluorine atoms in nilotinib formed a hydrogen bond with the hydroxyl group of residue Ser119. Moreover, a strong arene-cation interaction was observed between the pyrimidine ring of nilotinib and residue Arg106. For the CYP3A4-sunitinib complex, a similar arene-cation interaction between the benzene ring of indole in sunitinib and residue Arg106 was also observed (Fig. 4B), but no hydrogen bond was found in the complex. In the CYP2C8-nilotinib complex (Fig. 4C), the fluorine atoms in nilotinib also contributed markedly to ligand binding by forming three hydrogen bonds with residues Thr240, Val237, and Asn204. In addition, another hydrogen bond between the amino hydrogen of methylimidazole in nilotinib and the carbonyl oxygen of residue Asp293 was observed. In contrast, sunitinib formed one hydrogen bond between its carbonyl oxygen and the hydroxyl hydrogen of residue Ser103 and was located far from the heme center of CYP2C8 as compared with nilotinib (Fig. 4D).
The inhibitor–protein interaction diagram. (A) CYP3A4-nilotinib complex. (B) CYP3A4–sunitinib complex. (C) CYP2C8–nilotinib complex. (D) CYP2C8–sunitinib complex.
Spectral Analysis of Inhibitor and CYP Enzyme Binding.
To characterize the binding types between kinase inhibitors and P450 enzymes, absorbance differences of CYP3A4 or CYP2C8 in the presence and absence of kinase inhibitors were recorded from 350 to 500 nm using a UV-visible spectrophotometer. As summarized in Table 4, ketoconazole, a typical type II inhibitor, displayed characteristic binding spectra for both CYP3A4 and CYP2C8. For CYP2C8, most of these kinase inhibitors exhibited characteristic type II binding spectra, except bosutinib, dasatinib, and sunitinib. For CYP3A4, a majority of kinase inhibitors exhibited type II or type II-like binding spectra, except afatinib, erlotinib, lapatinib, and sunitinib for which a defined spectrum could not be obtained due to weak binding. Interestingly, sorafenib was the only inhibitor that displayed a type I binding with CYP3A4. Based on the available Ks values (Table 4), a good correlation was observed between Ks and Ki, respectively, for CYP3A4 (r2 = 0.807) and CYP2C8 (r2 = 0.950).
UV-visible spectral differences of CYP3A4 and CYP2C8 in the presence of selected kinase inhibitors
Spectral analysis was performed out as described in Materials and Methods. The binding type was defined based on Soret maximum and trough absorbance (Dahal et al., 2012): type I, maximum 385–390 nm, trough 415–420 nm; type II, maximum 420–435 nm, trough 390–405 nm. Ketoconazole, a typical type II inhibitor, was used as a positive control for system validation.
Prediction of Paclitaxel AUC Changes in the Presence of Kinase Inhibitors.
The potential of drug-drug interactions between paclitaxel and selected kinase inhibitors was examined by predicting AUC changes in paclitaxel exposure (the victim drug). First, the interactions were predicted based on reversible inhibition of both CYP2C8 and CYP3A4 pathways by kinase inhibitors, and the results are summarized in Table 5. By using the simple (1+I/Ki) values, some of these selected kinase inhibitors could elicit significant drug-drug interactions with paclitaxel by inhibiting either the CYP2C8 or CYP3A4 pathway, such as nilotinib, pazopanib, and sorafenib for CYP2C8, and bosutinib, nilotinib, and pazopanib for CYP3A4.
Prediction of drug-drug interactions between paclitaxel and kinase inhibitors based on reversible inhibition
Further analysis using the static equation (eq. 1) incorporating CYP2C8 and CYP3A4 parallel pathways but without the consideration of paclitaxel EH suggested that nilotinib, pazopanib, and sorafenib could cause marked drug-drug interactions (more than 6-fold increases in AUC) with paclitaxel (Table 5). Using eq. 3 and with the consideration of paclitaxel EH (0.78) and parallel pathways, the predicted drug-interactions dramatically decreased for nilotinib, pazopanib, and sorafenib. In this case, the maximal drug-drug interaction predicted was about 3.8-fold that could be caused by nilotinib, a potent inhibitor for both CYP2C8 and CYP3A4 pathways (Table 5). Simulations with varying EH values of paclitaxel revealed that the magnitude of interactions predicted for these kinase inhibitors was reduced with increasing EH. For example, nilotinib could cause more than 12-fold interaction when a theoretical EH of 0.1 was used for prediction. In contrast, the fold change in AUCs decreased to 2.3-fold using a theoretical paclitaxel EH of 0.9 (Table 5). A similar trend was observed for other kinase inhibitors such as lapatinib, pazopanib, and sorafenib, suggesting that the EH of paclitaxel might have a profound effect on the prediction accuracy. Although clinical interaction data are limited between paclitaxel and the combining kinase inhibitors, it appears that the equation (eq. 3) described by Kirby and Unadkat (2010) achieved a better performance in predicting drug-drug interactions mediated by reversible inhibition by those kinase inhibitors, if a 2-fold rule was applied (Guest et al., 2011).
Because most of those kinase inhibitors have been shown to be time-dependent inactivators of CYP3A4, their interaction potential with paclitaxel was predicted based on reversible inhibition of CYP2C8 and time-dependent inactivation of CYP3A4 simultaneously. For this purpose, eq. 4 with the consideration of paclitaxel EH (0.78) was used, as eq. 2 (ignoring EH) resulted in a marked overprediction as described earlier (data not shown). As shown in Table 6, an increased propensity of drug-drug interactions was noted when time-dependent inactivation of CYP3A4 was incorporated for prediction, as is evident by the larger AUC changes. As stated previously, HLMs from some donors exhibited higher CYP3A4 activities, leading to a higher fm,3A4 in paclitaxel metabolism; thus, we examined the effect of fm,3A4 on drug interactions.
Prediction of drug–drug interactions between paclitaxel and kinase inhibitors by incorporating time-dependent inactivation of CYP3A4
As expected, increases in CYP3A4 contribution to paclitaxel metabolism (fm,3A4 from 0.1 to 0.9) resulted in bigger AUC changes. For nilotinib, a marked interaction with paclitaxel could be anticipated as the compound is a potent reversible inhibitor of CYP2C8 and a potent time-dependent inactivator of CYP3A4. The low interaction potential predicted for axitinib with paclitaxel could be due to its low plasma concentrations (Martin et al., 2012), even though the drug was a potent CYP2C8 and CYP3A4 inhibitor based on in vitro data. It appears that ignoring the EH of paclitaxel (Table 5) and the higher contribution of CYP3A4 metabolism (higher fm,3A4, Table 6) results in overprediction of the interactions between paclitaxel and those kinase inhibitors.
Discussion
The activity of individual cytochrome P450 enzymes in HLMs varies markedly due to factors such as the organ donors’ genetic background, health condition, and medication history. With respect to CYP2C8 and CYP3A4, different ratios in the formation of 6α-hydroxypaclitaxel (CYP2C8) versus p-3′-hydroxypaclitaxel (CYP3A4) have been reported (Sonnichsen et al., 1995). In our present study, the formation of 6α-hydroxypaclitaxel was about 9 times higher than that of p-3′-hydroxypaclitaxel based on the observed Vmax/Km ratio, indicating that CYP2C8-mediated paclitaxel hydroxylation was more predominant in the current batch of HLMs. The predominance of 6α-hydroxypaclitaxel formation, up to 13-fold higher as compared with p-3′-hydroxypaclitaxel, was also observed in some individual livers (Václavíková et al., 2003; Taniguchi et al., 2005). In vivo, the level of 6α-hydroxypaclitaxel was about 4 times higher than that of p-3′-hydroxypaclitaxel in bile and urine samples, suggesting that CYP2C8 played a major role in paclitaxel metabolism in human (Monsarrat et al., 1998). Recent reports indicate that paclitaxel-induced neurotoxicity is correlated with CYP2C8 activity and the level of 6α-hydroxypaclitaxel (Leskelä et al., 2011; Hertz et al., 2012, 2013). In addition, CYP3A4*22 carriers with decreased enzyme activity are associated with an increased risk of neurotoxicity (de Graan et al., 2013). These findings suggest that P450-mediated paclitaxel metabolism and possibly specific metabolites are important factors affecting its clinical efficacy, thus highlighting the clinical relevance of drug-drug interactions and associated changes in metabolic clearance for paclitaxel-based combination therapies.
Inhibitory potency of a given drug against individual P450 enzymes is often characterized based on specific probe reactions (Bjornsson et al., 2003; Huang et al., 2007). Although data obtained using this approach are valuable for the characterization of drug interaction potentials and the design of adequate clinical investigations, it is also important to know whether a kinase inhibitor (often in chronic administration) will interfere with the metabolic clearance of the combining agent such as paclitaxel. For this purpose, our present study was undertaken to evaluate the inhibition of CYP2C8- and CYP3A4-mediated paclitaxel metabolism simultaneously by selected kinase inhibitors. Based on the observed Ki values, a pathway-dependent reversible inhibition was observed against CYP2C8- or CYP3A4-mediated paclitaxel metabolism, suggesting that some kinase inhibitors could selectively alter metabolic pathways of paclitaxel. The alteration of CYP2C8 and/or CYP3A4 activity may lead to changes in metabolite ratios, thus affecting the efficacy and safety outcomes of paclitaxel-based combination therapies, as paclitaxel-induced neurotoxicity has been correlated to CYP2C8 or CYP3A4 activity (Leskelä et al., 2011; Hertz et al., 2012; de Graan et al., 2013). Inhibition of CYP2C8- and/or CYP3A4-mediated paclitaxel metabolism in HLMs also has been reported for efflux-reversing agents and phenolic compounds (Desai et al., 1998; Václavíková et al., 2003).
With respect to time-dependent inhibition, the selected kinase inhibitors did not show marked effects against CYP2C8 with the exception of afatinib, erlotinib, gefitinib, lapatinib, and sorafenib, for which a modest time-dependent inhibition was noted. Further investigation of this finding is under way to delineate CYP2C8 inactivation mechanisms. For the CYP3A4 pathway, time-dependent inhibition was observed for the majority of selected kinase inhibitors, consistent with literature reports where midazolam is used as a probe substrate (Li et al., 2009a,b, 2010; Teng et al., 2010; Kenny et al., 2012). For some of those kinase inhibitors, inactivation mechanisms were also investigated (Teng et al., 2010; Takakusa et al., 2011). As observed for axitinib in our present study, a majority of those compounds have the potential to form reactive intermediates during P450 catalysis, as evident by the formation of glutathione adducts (Kenny et al., 2012). It thus appears that enzyme inactivation is a common mechanism that may be associated with CYP3A4-mediated drug interactions caused by small molecule kinase inhibitors.
The kinase inhibitors investigated herein represent different core structures and could be categorized into quinazoline, quinoline, aminopyrimidine, aminothiazole, and others based on the binding mode with the ATP-active site of different kinases. In the docking simulation with CYP3A4 and CYP2C8, a moderate correlation was found between the Glide docking scores and the experimental Ki values of CYP3A4, but the docking scores and the experimental Ki values of CYP2C8 showed a weak linear correlation. These results suggest that there is need for further improvements in the characterization of interactions between the model inhibitors (08Y and MTK) and respective P450 enzymes.
Spectral analysis between kinase inhibitors and CYP3A4 or CYP2C8 can provide insight on the observed inhibitory effects. These kinase inhibitors contain heterocyclic nitrogen atoms that may bind to the heme-iron of P450 enzymes, resulting in enzyme activity inhibition. Our study revealed that most of the kinase inhibitors display characteristic spectra of type II binding to CYP2C8 and/or CYP3A4, as exemplified by nilotinib (Table 4). For most of these type II binders, strong inhibition was observed, with a few exceptions such as afatinib for CYP2C8. It is interesting to note that the strong CYP2C8 inhibitor bosutinib did not show the type II binding spectrum. Comparison of available Ks and Ki values reveals a good correlation for both CYP3A4 and CYP2C8. As shown previously for compounds that contain heterocyclic nitrogen, the binding types and affinity can be affected by the availability of such nitrogen for iron coordination due to steric hindrance and ring substitution (Chiba et al., 2001; Dahal et al., 2012). As such, ongoing efforts aim to characterize the contribution of various interactions (iron coordination, hydrogen binding, hydrophobic interaction, etc.) to the observed inhibitory effects.
Prediction of drug-drug interactions using in vitro data has been extensively explored using both static and dynamic models, and considerable success has been achieved (Ito et al., 2005; Obach et al., 2006; Lu et al., 2008; Fahmi et al., 2008; Rowland Yeo et al., 2010). For intravenously administered drugs, it has been suggested that this type of modeling can be used for prediction assuming a very low hepatic EH (Kirby and Unadkat, 2010). In our study, the AUC ratios of paclitaxel (an intravenous drug) are predicted by ignoring the hepatic EH of paclitaxel as well as by considering EH (Kirby and Unadkat, 2010). When compared with available clinical reports on pharmacokinetic interactions between paclitaxel and kinase inhibitors (Table 1 and Table 5), the current analysis shows that the EH of paclitaxel had a marked impact on the fold of predicted interactions, as a lower extraction ratio; ignoring the EH is linked to overprediction for several kinase inhibitors. Furthermore, overprediction is also noted when the time-dependent inhibition component of CYP3A4 is incorporated, which is in turn affected by the fm,3A4 of paclitaxel (Table 6). Overprediction of drug–drug interactions has been reported for CYP3A4 inactivation by various kinase inhibitors (Kenny et al., 2012). For experimentally determined kinetic parameters (such as Ki), nonspecific binding and albumin tend to decrease “effective concentrations” of some drugs and lead to overestimation of parameters obtained from HLM incubations (Margolis and Obach 2003; Wattanachai et al., 2011; Nagar and Korzekwa 2012). The decreased inhibitory potency due to overestimation of Ki values may result in underprediction of drug-drug interaction potential, a phenomenon was not seen during our analysis. In our study, a reasonably accurate prediction was obtained using Ki values generated with nominal inhibitor concentrations in HLMs, inhibitor plasma Cmax reported in the literature, and the incorporation of the EH of paclitaxel (the victim drug).
Clinically significant drug interactions between paclitaxel and kinase inhibitors based on AUC changes have not been reported, even though paclitaxel is frequently combined with various kinase inhibitors for the treatment of a variety of tumor types. From the limited clinical data and current prediction, it appears that the likelihood of a strong interaction is relatively low for a majority of kinase inhibitors tested. Because actual drug-drug interactions in the clinical setting depend on multiple factors such as dose levels, dose regimen (timing of drug administration), and the patient’s genetic background, potential drug-drug interactions between paclitaxel and kinase inhibitors (such as nilotinib) via strong CYP2C8 and/or CYP3A4 inhibition should not be ignored. Although pharmacokinetic drug interactions are often described by AUC changes in parent drugs, exposure alteration in key metabolites is also worthwhile to describe. Because changes in the CYP3A4 (de Graan et al., 2013) and/or CYP2C8 (Hertz et al., 2012, 2013) metabolism of paclitaxel are correlated with an increased risk of neurotoxicity, differential metabolism (or metabolite ratio) in the presence of an inhibitor may be clinically relevant. Therefore, the availability of in vivo information on pharmacokinetic changes in parent drugs as well as key metabolites would be valuable not only to confirm the utility of predictive models but also to define the underlying mechanisms.
In conclusion, pathway-dependent inhibition (CYP2C8 versus CYP3A4) and time-dependent inhibition (CYP3A4) of paclitaxel metabolism by selected kinase inhibitors were observed. Molecular docking simulations revealed that potent inhibition of CYP2C8 and CYP3A4 by nilotinib can be explained by the strong binding between nilotinib and respective enzymes. In addition, the type II binding to underlying P450 enzymes was evident for the majority of kinase inhibitors. With the consideration of EH of the victim drug paclitaxel, a reasonably accurate prediction of drug-drug interactions between paclitaxel and kinase inhibitors is achieved. Although clinically significant drug interactions have not been reported, the strong in vitro inhibition of CYP2C8- and CYP3A4-mediated paclitaxel metabolism by some kinase inhibitors warrants further evaluation. In particular, alteration in metabolite formation and its relationship to paclitaxel-induced neurotoxicity needs to be addressed. It can be anticipated that further investigations in vivo will lead to safer and more effective use of paclitaxel-based combination therapies.
Acknowledgments
The authors thank Xingxing Diao and Dr. Xiaoyan Chen at the Shanghai Institute of Materia Medica for technical assistance with the identification of axitinib-glutathione adducts using ultra-performance liquid chromatography quadruple time-of-flight accurate mass spectrometry.
Authorship Contributions
Participated in research design: Y. Wang, He, Zhang.
Conducted experiments: Y. Wang, M. Wang, Qi, Li, He, Pan, Hou.
Performed data analysis: Y. Wang, M. Wang, Li, Zhang.
Wrote or contributed to the writing of the manuscript: Y. Wang, Hou, Zhang.
Footnotes
- Received July 19, 2013.
- Accepted January 29, 2014.
This work was supported by a grant from Soochow University, Suzhou, People’s Republic of China [Grant Q413200711].
↵
This article has supplemental material available at dmd.aspetjournals.org.
Abbreviations
- AUC
- area under the curve
- CE
- collision energy
- CLH
- hepatic clearance
- EH
- hepatic extraction ratio
- fhep
- fraction of clearance subject to hepatic blood flow limitation
- fm
- fraction metabolized
- HLM
- human liver microsomes
- kdeg
- enzyme degradation rate constant
- Ki
- reversible inhibition constant
- KI
- inhibitor concentration that results in half-maximal enzyme inactivation
- kinact
- inactivation rate constant
- Ks
- spectral dissociation constant
- LC-MS/MS
- liquid chromatography–tandem mass spectrometry
- MTK
- montelukast
- P450
- cytochrome P450
- P-gp
- P-glycoprotein
- 08Y
- bromergocryptine
- Copyright © 2014 by The American Society for Pharmacology and Experimental Therapeutics